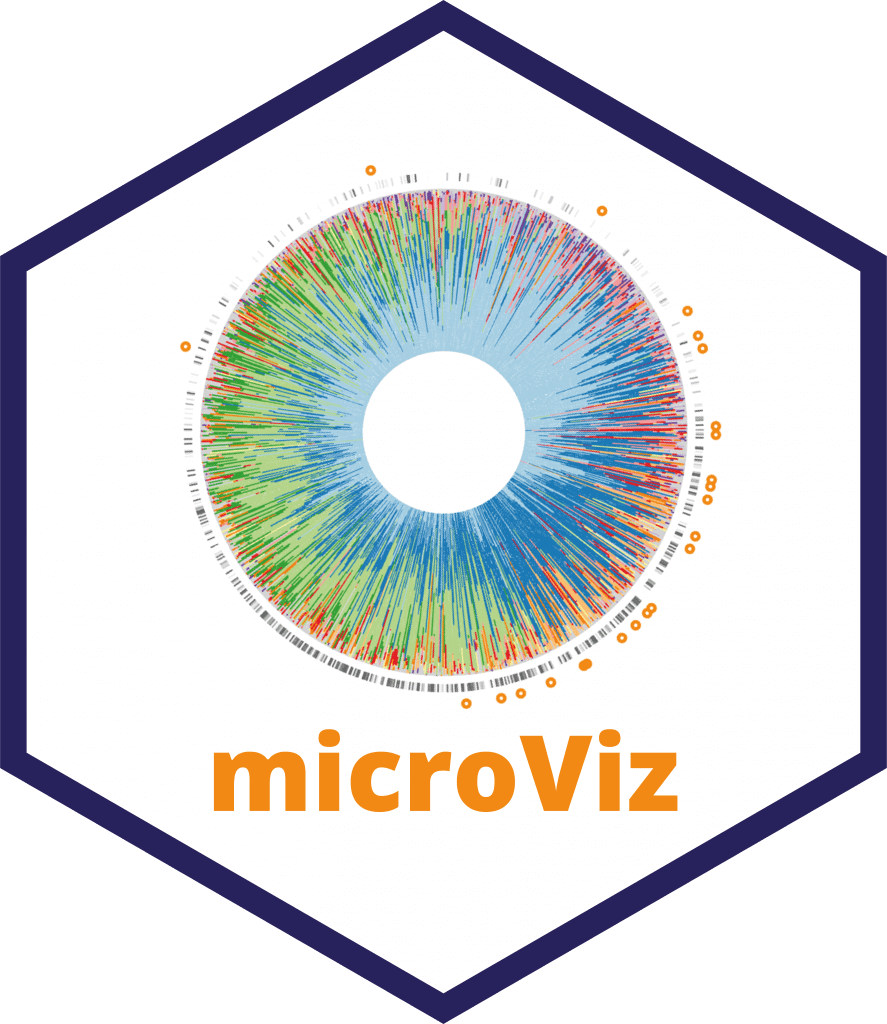
Extract statistics from taxatree_models or tax_model output
Source:R/taxatree_models2stats.R
models2stats.Rd
Runs a function e.g. broom::tidy
on a list of models, i.e. the output of
taxatree_models
or tax_model
Usage
taxatree_models2stats(data, fun = "auto", ..., .keep_models = FALSE)
tax_models2stats(data, rank = NULL, fun = "auto", ..., .keep_models = FALSE)
Arguments
- data
psExtra with attached tax_models or taxatree_models list, or just the list of models
- fun
function to assist extraction of stats dataframe from models, or "auto"
- ...
extra arguments passed to fun
- .keep_models
should the models list be kept in the psExtra output?
- rank
string naming rank at which tax_model was run (needed if data is a list)
Functions
taxatree_models2stats()
: Extract stats from list or psExtra output of taxatree_modelstax_models2stats()
: Extract stats from list or psExtra output of tax_model
Examples
# This example is an abbreviated excerpt from article on taxon modelling on
# the microViz documentation website
library(dplyr)
data("ibd", package = "microViz")
# We'll keep only the Ulcerative Colitis and Healthy Control samples, to
# simplify the analyses for this example. We'll also remove the Species
# rank information, as most OTUs in this dataset are not assigned to a
# species. We'll also use `tax_fix` to fill any gaps where the Genus is
# unknown, with the family name or whatever higher rank classification is
# known.
phylo <- ibd %>%
ps_filter(DiseaseState %in% c("UC", "nonIBD")) %>%
tax_mutate(Species = NULL) %>%
tax_fix()
# Let's make some sample data variables that are easier to use and compare
# in the statistical modelling ahead. We will convert dichotomous
# categorical variables into similar binary variables (values: 1 for true,
# or 0 for false). We will also scale and center the numeric variable for
# age.
phylo <- phylo %>%
ps_mutate(
UC = ifelse(DiseaseState == "UC", yes = 1, no = 0),
female = ifelse(gender == "female", yes = 1, no = 0),
antibiotics = ifelse(abx == "abx", yes = 1, no = 0),
steroids = ifelse(steroids == "steroids", yes = 1, no = 0),
age_scaled = scale(age, center = TRUE, scale = TRUE)
)
lm_models <- phylo %>%
tax_fix() %>%
tax_prepend_ranks() %>%
tax_transform("compositional", rank = "Genus") %>%
tax_filter(min_prevalence = 0.1, use_counts = TRUE) %>%
taxatree_models(
type = lm,
trans = "log2", trans_args = list(zero_replace = "halfmin"),
ranks = c("Phylum", "Class", "Genus"),
variables = c("UC", "female", "antibiotics", "steroids", "age_scaled")
)
#> Proportional min_prevalence given: 0.1 --> min 7/67 samples.
#> 2025-04-14 11:43:16.088791 - modelling at rank: Phylum
#> 2025-04-14 11:43:16.248812 - modelling at rank: Class
#> 2025-04-14 11:43:16.969508 - modelling at rank: Genus
lm_stats <- lm_models %>% taxatree_models2stats()
# inspect the psExtra returned, now with taxatree_stats dataframe added
lm_stats
#> psExtra object - a phyloseq object with extra slots:
#>
#> phyloseq-class experiment-level object
#> otu_table() OTU Table: [ 68 taxa and 67 samples ]
#> sample_data() Sample Data: [ 67 samples by 19 sample variables ]
#> tax_table() Taxonomy Table: [ 68 taxa by 6 taxonomic ranks ]
#>
#> otu_get(counts = TRUE) [ 68 taxa and 67 samples ]
#>
#> psExtra info:
#> tax_agg = "Genus" tax_trans = "compositional"
#>
#> taxatree_stats dataframe:
#> 88 taxa at 3 ranks: Phylum, Class, Genus
#> 5 terms: UC, female, antibiotics, steroids, age_scaled
# inspect the dataframe itself
lm_stats %>% taxatree_stats_get()
#> # A tibble: 440 × 8
#> term taxon rank formula estimate std.error statistic p.value
#> <fct> <chr> <fct> <chr> <dbl> <dbl> <dbl> <dbl>
#> 1 UC P: Firmicutes Phyl… `P: Fi… 0.0430 0.455 0.0945 0.925
#> 2 female P: Firmicutes Phyl… `P: Fi… -0.297 0.324 -0.919 0.362
#> 3 antibiotics P: Firmicutes Phyl… `P: Fi… -0.793 0.440 -1.80 0.0763
#> 4 steroids P: Firmicutes Phyl… `P: Fi… -0.747 0.415 -1.80 0.0771
#> 5 age_scaled P: Firmicutes Phyl… `P: Fi… 0.144 0.183 0.788 0.434
#> 6 UC P: Bacteroide… Phyl… `P: Ba… -2.58 1.63 -1.58 0.120
#> 7 female P: Bacteroide… Phyl… `P: Ba… -1.01 1.16 -0.870 0.388
#> 8 antibiotics P: Bacteroide… Phyl… `P: Ba… 1.52 1.58 0.962 0.340
#> 9 steroids P: Bacteroide… Phyl… `P: Ba… -1.67 1.49 -1.12 0.266
#> 10 age_scaled P: Bacteroide… Phyl… `P: Ba… -0.340 0.655 -0.519 0.605
#> # ℹ 430 more rows
# keep the models on the psExtra object
lm_models %>% taxatree_models2stats(.keep_models = TRUE)
#> psExtra object - a phyloseq object with extra slots:
#>
#> phyloseq-class experiment-level object
#> otu_table() OTU Table: [ 68 taxa and 67 samples ]
#> sample_data() Sample Data: [ 67 samples by 19 sample variables ]
#> tax_table() Taxonomy Table: [ 68 taxa by 6 taxonomic ranks ]
#>
#> otu_get(counts = TRUE) [ 68 taxa and 67 samples ]
#>
#> psExtra info:
#> tax_agg = "Genus" tax_trans = "compositional"
#>
#> taxatree_models list:
#> Ranks: Phylum/Class/Genus
#> taxatree_stats dataframe:
#> 88 taxa at 3 ranks: Phylum, Class, Genus
#> 5 terms: UC, female, antibiotics, steroids, age_scaled
# you can adjust the p values with taxatree_stats_p_adjust
# you can plot the results with taxatree_plots